Automated Integration and Piping routing for cooling systems
Automate cooling system integration and piping routing for EV, hybrid, and ICE vehicles with AI. Optimize battery placement, boost design speed, and cut risks.
Choosing the right cooling system architecture is a critical step in the development of ICE, hybrid, and electric vehicles. Early decisions about battery placement, power electronics positioning, and cooling efficiency can have major impacts on project costs, performance, and timelines.
In this use case, we demonstrate the use of a customized library developed within the Dessia platform to automate the exploration of design options. By combining 3D layout optimization with automated routing, the AI-Apps help engineers identify the best cooling architectures faster, with greater accuracy, and with broader exploration of design possibilities.
This approach strengthens decision-making during the crucial preliminary design phases, while significantly reducing manual effort and design risks.
1. Context and challenges
During the preliminary phases, engineers are often faced with complex choices involving multiple assumptions, with the goal of determining the optimal solution. This solution is then handed over to design offices for the detailed design phase and to conduct the necessary consultations with external suppliers. This stage is crucial, as it commits the company to strategic decisions that cannot be reversed later on. For example, engineers must decide where to place the various battery modules and what cooling architecture to adopt, which requires careful decision-making.
In this document, we will explain how customized AI-Apps developed using the Dessia software solution can assist architects and designers in making informed decisions regarding a vehicle’s cooling architecture. The cooling architecture studied here includes two batteries (high-voltage and low-voltage), a power electronics unit, a heat exchanger, a radiator, and the powertrain.
The objective is to determine the best positioning for the two batteries and the power electronics unit while respecting the placement constraints of these three components.
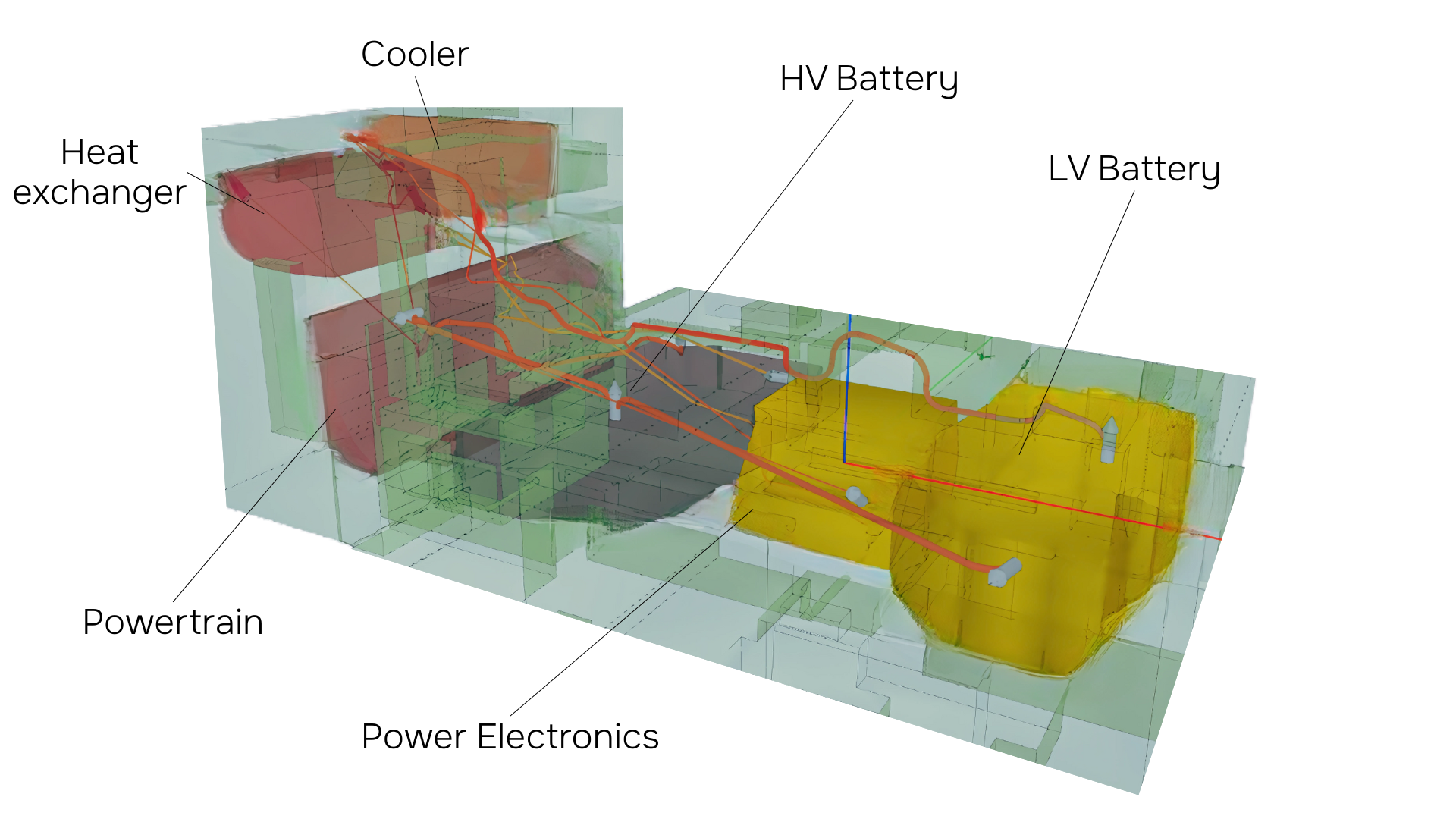
2. Engineering problem formalization
The available packaging volume for the electric vehicle is represented by the following volume. This was obtained by simplifying a CAD model of a real vehicle using basic geometric elements. To create this simplified elementary volume, two approaches are possible: either by using the Dessia SDK, which allows automatic construction of this volume from a real CAD model, or by manually creating it using standard CAD software.
It can be assumed that the locations of the heat exchanger, radiator, and powertrain have already been defined. Thus, the following volume represents the search space where the two batteries and the power electronics unit are to be positioned.
To carry out our study effectively, we will use two separate AI applications. The first is responsible for exploring all possible configurations for positioning the batteries and power electronics (layout), while the second automatically maps out the various fluid circuits (piping).
3. Layout library
To position the various components, the Dessia software provides a library that uses a method based on volume discretization using voxels, followed by the creation of clusters representing possible positions for these components. The image below shows the power electronics unit discretized using voxels (3D pixels). This discretization aims to identify the most likely areas for placing the power electronics within the available volume. These likely areas are then used as initial conditions for a more precise placement algorithm (genetic algorithm), in order to generate a large number of possible 3D positions for these components.
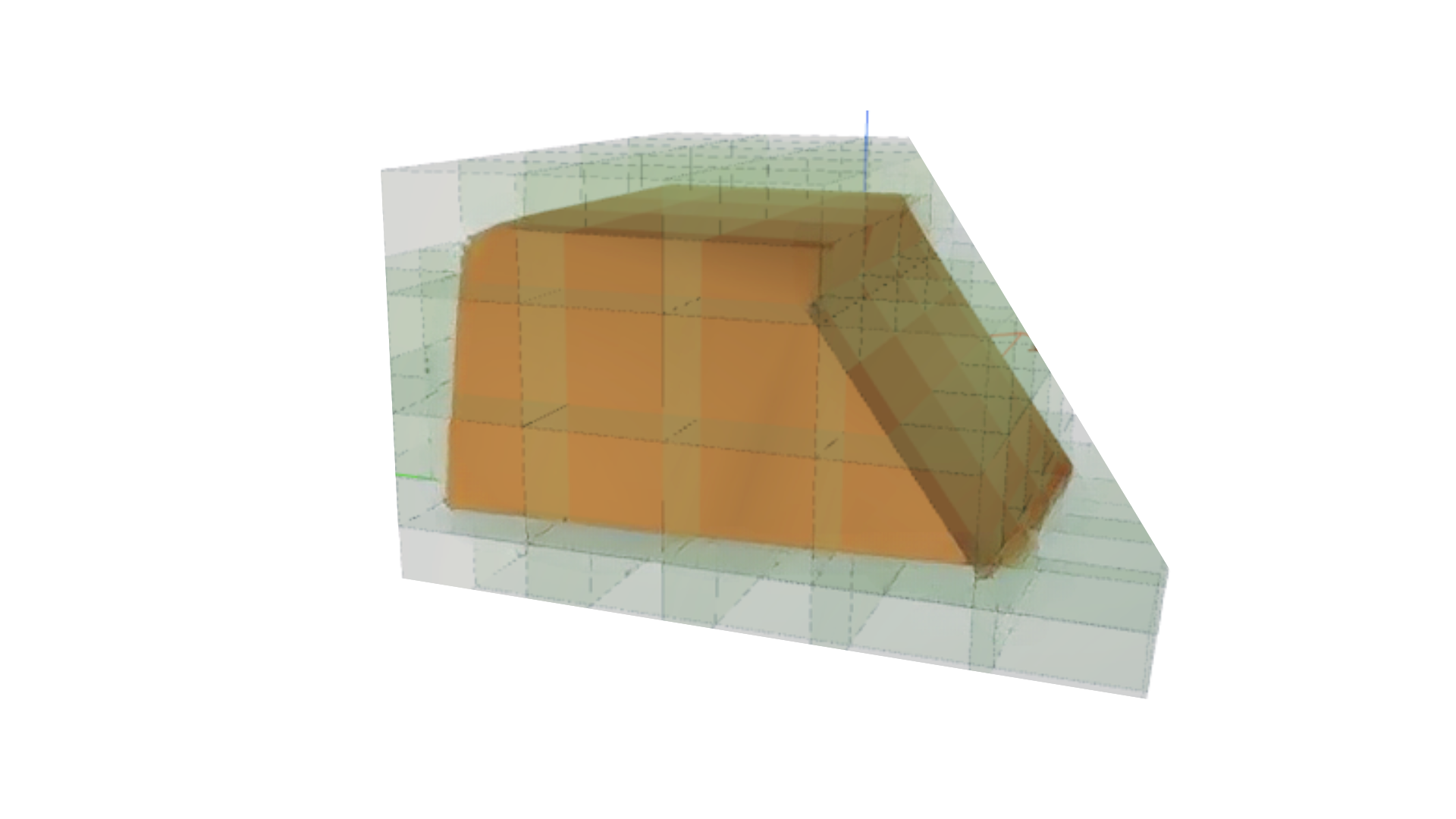
In the image below, a customized AI-App has generated all possible positions for the power electronics unit. A classification was performed using a clustering method, where each color represents a different cluster. In this example, five clusters were used to represent all possible positions of the power electronics. The red cluster corresponds to solutions where the power electronics is located at the far right of the packaging volume, while the green cluster represents solutions where it is placed at the far left.

An automated approach was used to position the various components, namely the two batteries and the power electronics unit. A large number of possible configurations (3,000 architectures) were generated by combining all possible positions of these components, obtained through voxel discretization and clustering classification. Three different architectures were selected: the first places the high-voltage battery on the right side, the second positions it in the center, and the third places it on the left side.
%20and%20solution%202%20(right).png)
4. Routing library
From each 3D solution and the functional diagram of the desired connections between components, a customized AI-App -built from a routing library- automatically traces all the 3D pipes. Dessia’s automatic routing algorithm relies on a mesh that is generated automatically, followed by a search for the shortest path between two components, constrained by business rules. More specifically, each component has a number of ports representing the connection points on the component. The algorithms thus search for the shortest path between two different ports.
The following images represent 3 optimal solutions . These are initial functional layouts, and pipe interferences are not considered at this stage of the study. Each solution is generated in a few minutes, and all 3,000 architectures are studied in 3 hours.

Conclusion
Thanks to the two AI-Apps developed with the Dessia software solution and its libraries, an automated process for comparing solutions has been augmented in order to select the optimal architecture for the cooling system. Final cost using a linear function has been set that takes into account the piping diameter and the curvilinear length.
As a result, development time has been significantly shortened, while the number of solutions has increased due to designers being able to make more informed decisions.
Keep exploring - Discover more case studies
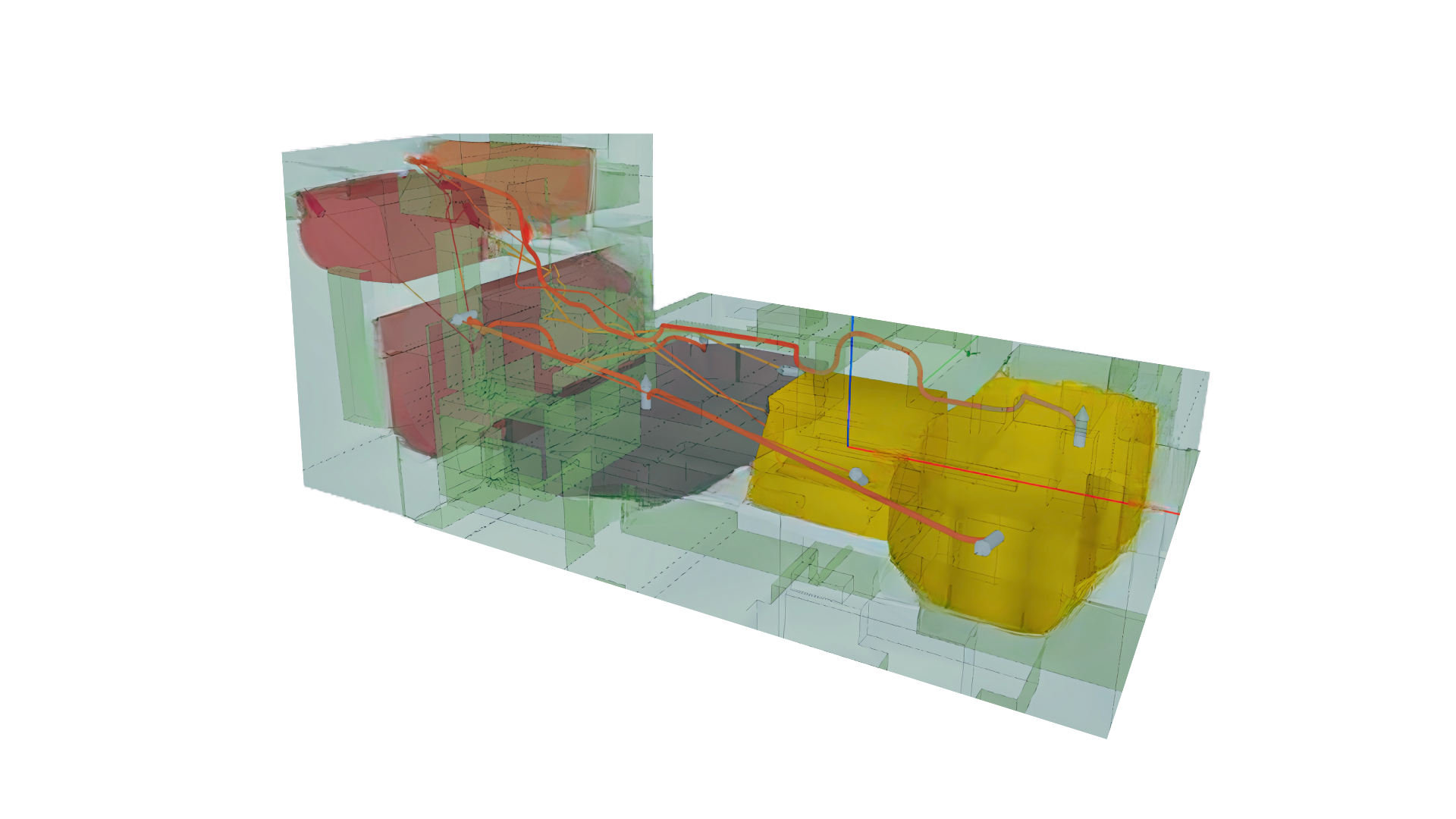
System Architecture Generation
min reading
